Data, Innovation and Community Engagement Distinguished Lecture Series
Data and Software Drivers in Computational Sensing
Speaker: Stephan Robila Ph.D.
Computational sensing is the process of extraction, analysis and use of knowledge about the sensed phenomena. In computational sensing we aim to address sensing problems through interdisciplinary approaches that include instrument design, phenomena modeling, simulation and experimentation, and use of high-performance computational environments. In this talk I will provide an overview of my work that included research on feature extraction and parallel and distributed computing and then discuss how some of these approaches can be accelerated through the development of integrated scientific workflows. A data processing workflow is the process of how data are collected, aggregated, validated, and analyzed/transformed. In the last decades, given the exponential growth in data collection, the availability of significant compute resources and the increase in complexity and diversity of scientific approaches, the concept of scientific workflow has been more precisely aligned with the use of cyberinfrastructure (CI). It is now defined as computer supported processes that enable scientific discovery at scale. Seeking to move from creating individual workflow for a specific problem to the more general concept of integrated workflow design, development and deployment (that would target classes of problems or address a set of data and compute scenarios) I am currently investigating how components in the scientific processing pipeline impact overall workflow design. The presentation is anchored in two research directions: remote sensing and computing sustainability. For remote sensing, I provide an overview of my previous research with hyperspectral data and show how future frameworks will be able to ingest vast amounts of data in seeking answers for problems at large scale. For computer sustainability I discuss how energy consumption has been measured for large systems hosted in data centers and present the case for an individual site. Then I discuss how data can be combined to build a global estimate for computer energy use and how such estimates can be updated as the new technologies are introduced.
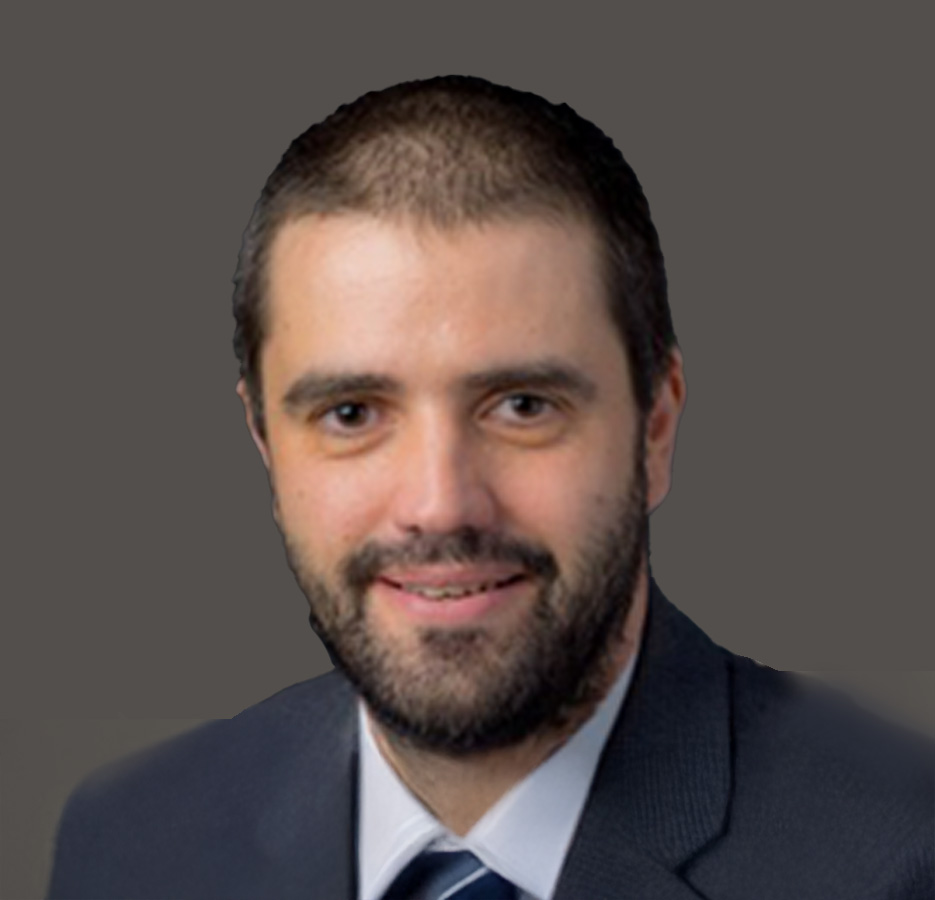